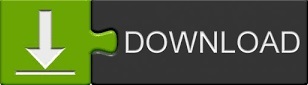
The prediction task was to classify which patients were going to enter an episode of acute hypotension in the forecast window of one hour after the last entry in the data set. It consists of 1–6 days of high-frequency physiological data from patients in an Intensive Care Unit (ICU). To compare results, we used one of the data sets from previous work which came from the Physionet Challenge. We compare the best results in the multivariate domain to the results we achieved in the univariate domain with this new approach to test its potential effectiveness. In this case, the edit cost probabilities are learned by a stochastic Finite-State Transducer (FST).
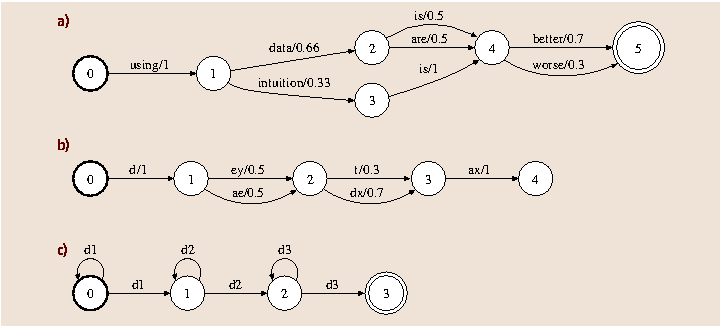
#FINITE STATE AUTOMATA STOCHASTIC SERIES#
We are using Stochastic Edit Distance on a concatenated symbolic representation of the time series to classify a physiological data set for an acute episode of hypotension. This paper represents work in the univariate time series domain which we are examining for application to the multivariate domain. The results demonstrated that the multivariate representations outperformed univariate ones for the purpose of predicting the targeted outcome. Similarity was measured by converting the data sets into the indicated representations and then classifying the data using the Nearest Neighbor algorithm. Two data sets were examined in each domain for a total of six different data sets. The representations were tested in three distinct data domains: field-motion capture data, robot sensor data, and ICU data. In related work, four multivariate time series representations were examined to serve as compressed representation of high frequency physiological data from Intensive Care Units: Stacked Bags-of-Patterns, Multivariate Bags-of-Patterns, Multivariate Piecewise Dynamic Time Warping and Ensemble Voting with Bag-of-Patterns. Many are recognizing the importance of analyzing this data as a multivariate temporal representation by creating multivariate probabilistic models or temporal abstractions from electronic health records or creating multivariate structures that are similar to those in other domains such as convolutional neural networks or imaging. Recently, however, there has been interest in storing and analyzing the high-frequency data using automated and semi-automated methods. Physicians make life-saving decision based on this lower-frequency data. Instead, a lower-frequency version of this data is stored in an electronic health record after validation by a medical provider at the rate of once every 15 min to once every several hours.
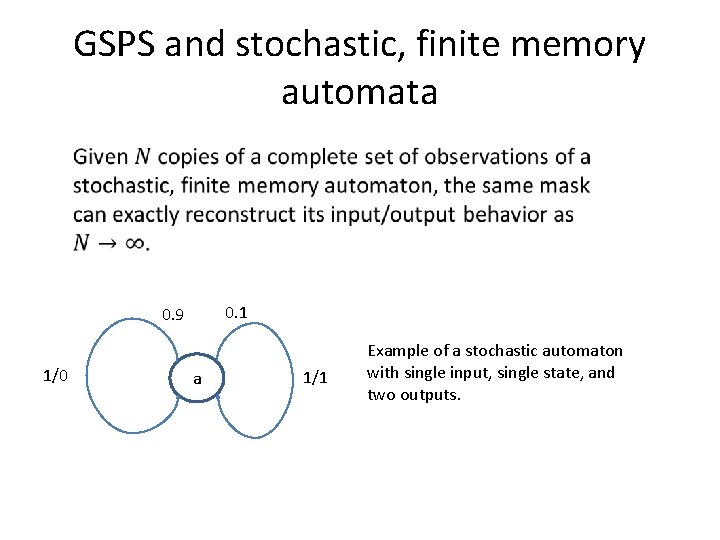
These measurements are displayed on a monitor for a few seconds as a collection of univariate time series and then lost to further analysis. Current methods for measuring the well-being of a patient in the intensive care unit (ICU) acquire a patient’s vital signs data at rates that are difficult for a human to analyze (60–500 Hz).
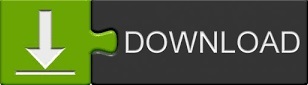